A Community of “Accelerators”
In June of 2021, the Therapeutics Parallel Analysis (TxPA) work group of the COVID-19 Evidence Accelerator paused to conduct a retrospective or “retro”. For those of you who are unfamiliar with the expression, a retro is an opportunity to review the work of a previous cycle or “sprint” in Agile terminology. A retro allows the Evidence Accelerator community the opportunity to step back and see how far we have come, what went well, and where we could make improvements.
The retro revealed a few themes which we plan to discuss in some detail in a series of blog posts. This first article examines the development of “Community” in the Evidence Accelerator.
Our first TxPA workstream focused on the question of hydroxychloroquine use in COVID-19 patients concluded in the fall of 2020 resulting in our first publication. The TxPA work group has made significant progress since the Fall of 2020, focusing on our two current workstreams: Natural History of Coagulopathy and Remdesivir. The questions that we chose to tackle were developed collaboratively between FDA and the broader Evidence Accelerator community, specifically:
- What is the natural history of coagulopathy in COVID-19 patients?
- Can we assess the effectiveness of remdesivir in hospitalized COVID-19 patients?
To address these questions, we worked with a community of volunteer groups known collectively as “Accelerators” with access to datasets, tools, and/or methodologies to build a robust and credible real-world evidence-base. Each group (Table 1) chose which question set they would like to tackle, based on their own interests and available resources. By working with groups willing to conduct these analyses in parallel using a common analytical plan, our goal was to gather quick insights into the management and treatment of patients with COVID-19.
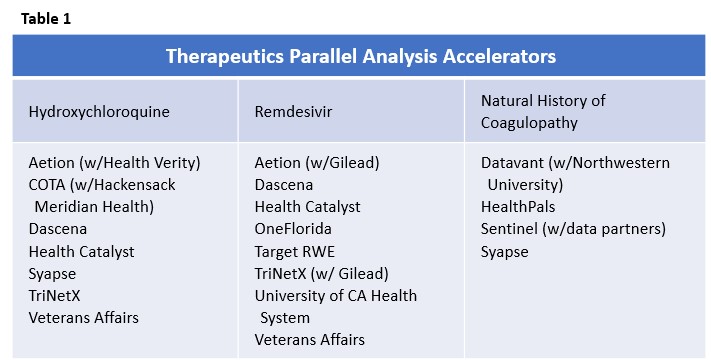
Each member of this newly formed community came to the group with different motivations; the wish to contribute to addressing the public health emergency, a desire to learn from peers regarding how they would tackle common problems, or an opportunity to showcase and sharpen their skills and expertise applying the most recent methods of real-world evidence. Below are some of the lessons learned from this process from the perspective of the community.
- Agreeing on a common analytical plan takes time. The TxPA community has substantial skills and expertise in designing and executing observational studies using real-world data. The teams authoring the analytical plans needed to iterate with others within the community to develop common plans, one for each question set. The newness of this disease, combined with the still-evolving methods and the varied level of expertise of those in the community meant that we needed to spend time to develop and refine these analytical plans and protocols. Over the course of several weeks, the groups reviewed multiple drafts and weighed the costs and benefits of different statistical approaches and methodologies.
- Each dataset presents its own unique challenges. By sharing experiences, the community was able to accelerate and share learnings amongst themselves. Groups with one type of dataset, e.g., electronic health records, appreciated hearing about the challenges and solutions of using different types of data, e.g., Chargemaster data or insurance claims. An example was medication use definitions, i.e., how did various groups identify the medications used in their analysis, whether medications of primary interest, e.g., remdesivir, or concomitant medications such as anticoagulants. While some groups used NDC codes, others used RxNORMS, or simple text searches. See our January 28, 2021 blog for more on this particular challenge.
- Contributors vary in how much time and resources they can devote. Along the way, some groups had to drop out or divert personnel as they realized that their other obligations precluded them from continuing. Lack of funding for the groups may have also been a factor for other groups, although other factors were certainly at play. Bandwidth concerns also impacted the speed with which results were generated and analyzed. As a result, the groups shared their results over a wider timeframe than originally anticipated. This was a particularly useful insight which we will need to consider when planning future research activities.
One reoccurring theme was the value of community, and having a venue for an exchange of ideas, knowledge, and even problems. In the fast-paced world of scientists responding to a fast-moving pandemic, access to fellow scientists doing different work, and approaching problems in varied ways was exciting, inspiring and invaluable. We will look to incorporate these and other lessons as we evolve the Evidence Accelerator and plan future research activities.
Additional lessons learned from the retro, grouped along other themes, will be explored in future posts. Stay tuned!