In parallel analysis workgroups, the Evidence Accelerator1 convenes experts in health systems research, regulatory science, data science, statistics, and epidemiology to rapidly collect information related to real-world studies of COVID-19. With leadership and input from the FDA, a foundational task of this parallel analysis is to understand what data are available to answer key COVID-19 questions of interest to public health. In the Evidence Accelerator, we crowdsource the relevance of the research questions initially developed by the FDA to get a sense of what, where, and when data are available. This exchange fuels the rapid development of master protocols to evaluate the safety and effectiveness of multiple treatments or multiple diagnostic tests in various sub-populations, both in parallel and in succession. Master protocols enable evaluation of the same question across different data systems using a parallel approach to analyses to quickly test reproducibility of results and evaluate data heterogeneity (see Figure 6.3).
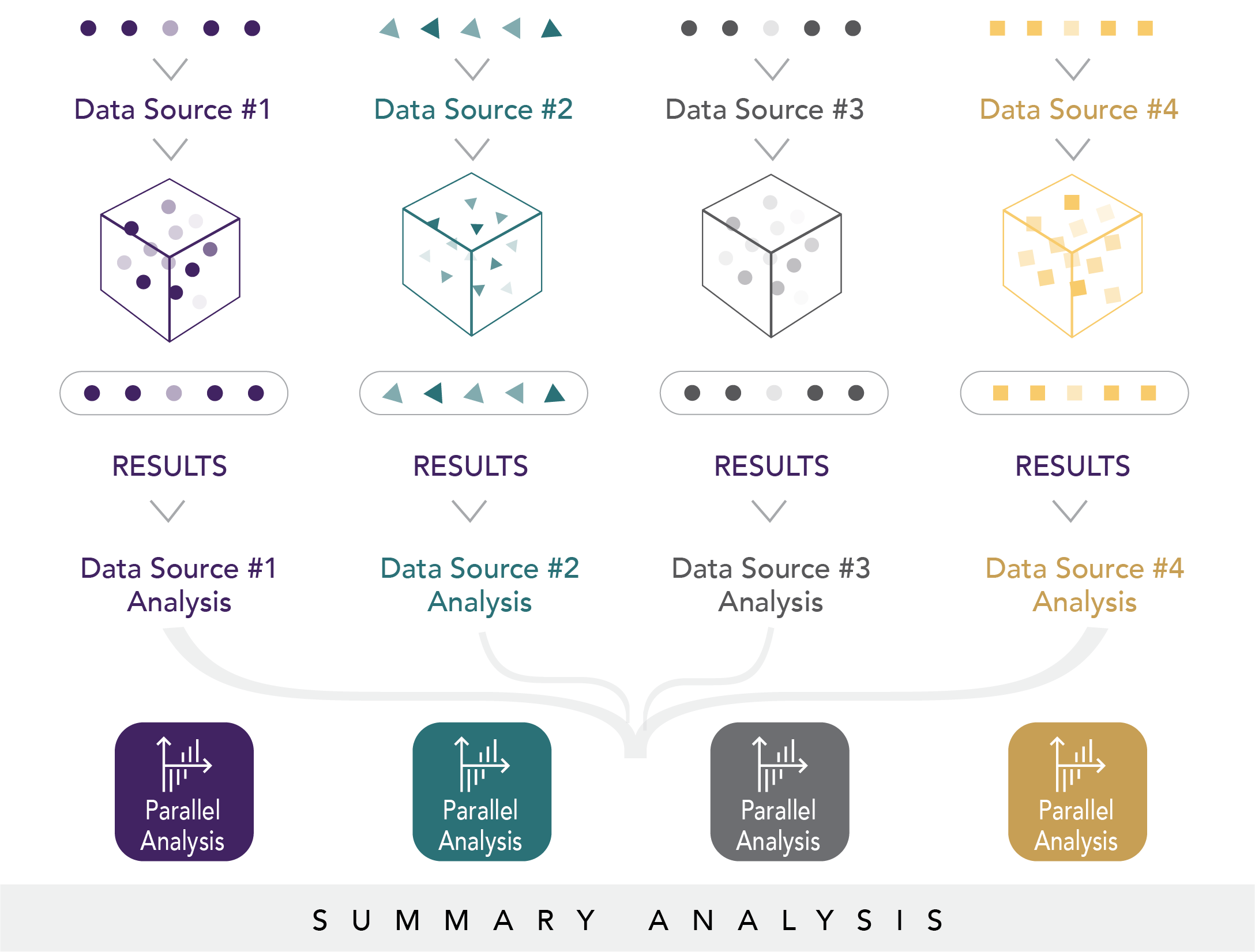
Figure 6.3. Parallel analysis
The parallel analysis groups work collaboratively to determine how data elements are being extracted and how they are being defined to operationalize a platform that can answer current questions, as well as to inform how research activities could be conducted in the future. Repeating analyses in parallel through collaborators using different analytical techniques and data sources helps strengthen findings and learnings. Through a set of community created core principles (Figure 6.4) that underpin all work efforts, the goal of the Evidence Accelerator parallel analysis is to help validate the role of real-world data as a tool for rapidly learning about patient characteristics, treatment patterns, and outcomes associated with management strategies for COVID-19.4
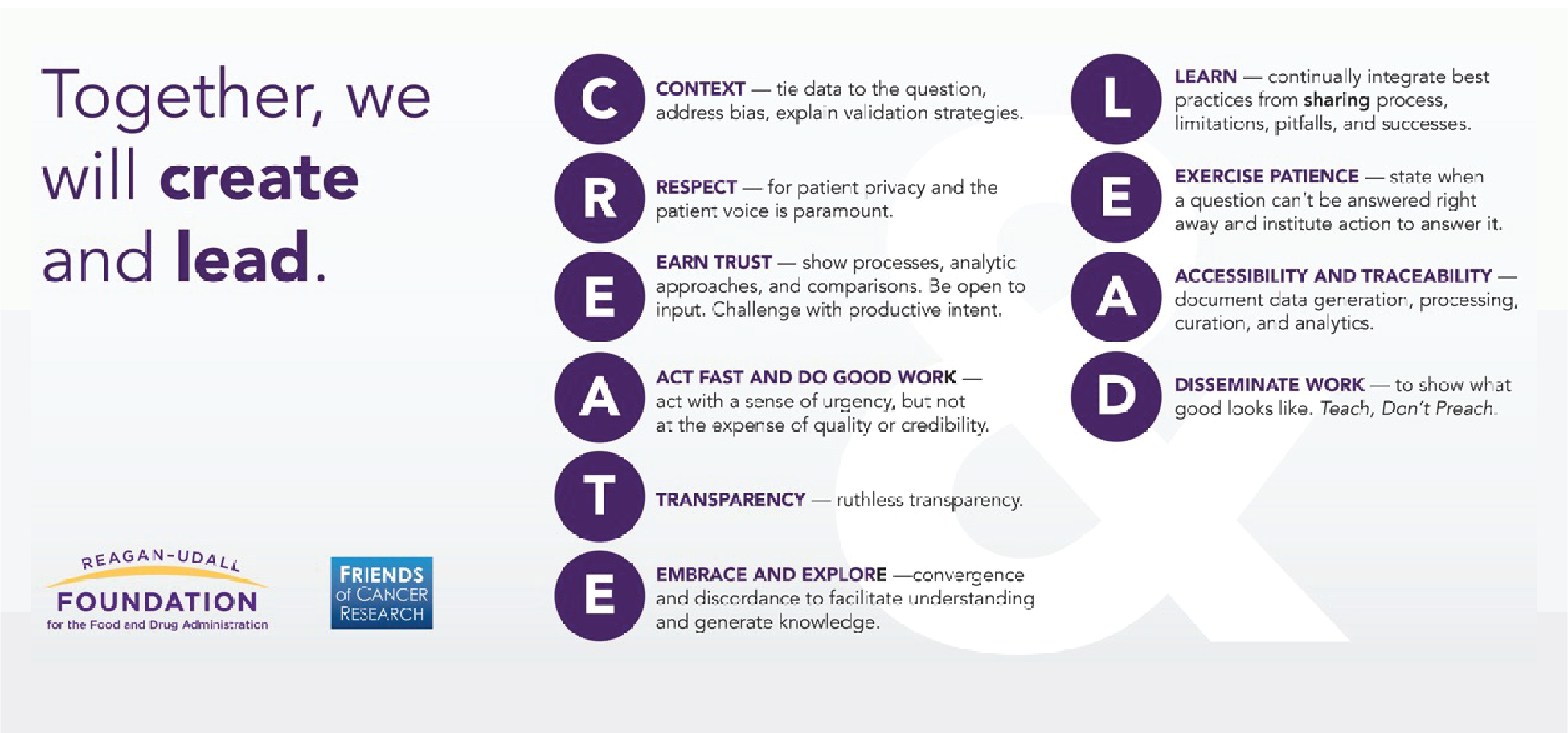
Figure 6.4. Community created core principles
Therapeutics and Diagnostics Evidence Accelerator Parallel Analysis Workgroups:
In close collaboration with the FDA, the Therapeutics Evidence Accelerator Parallel Analysis workgroup developed key specific COVID-19 research questions for multiple organizations and teams to address simultaneously. Initial activities include 1) rapidly revising a list of core data elements, 2) identifying those elements critical to answering the primary question, and 3) establishing uniform collection parameters. The Therapeutics Parallel Analysis workgroup has taken on 3 primary research areas to date: 1) effectiveness and safety of hydroxychloroquine (with and without azithromycin), 2) real-world safety and effectiveness of remdesivir for COVID-19, and 3) the natural history of coagulopathy in COVID-19 vs. influenza. This workgroup has met 65 times and presented 3 times to a broader audience. Results from the hydroxychloroquine project were published,5 and the results demonstrated across 7 databases that administration of hydroxychloroquine (with and without azithromycin) appeared to have no effect on time to mortality in hospitalized COVID-19 patients. More than 15 organizations have engaged in at least one of the parallel analyses (see Table 6.1).
The Diagnostic Parallel Analysis workgroup incorporates lessons learned from the Therapeutics Evidence Accelerator Parallel Analysis workgroup. It brings analytic partners together to address key research questions about diagnostic testing in parallel. This workgroup conducted the same 3 initial activities as the Therapeutics Parallel Analysis workgroup. These activities are described in detail in a master protocol.6 This workgroup operates collaboratively to figure out how data elements are being extracted and how they are being defined. This enables the group to put a platform into use that can answer current research questions.
Table 6.1. Organizations that have engaged in at least one of the parallel analyses.
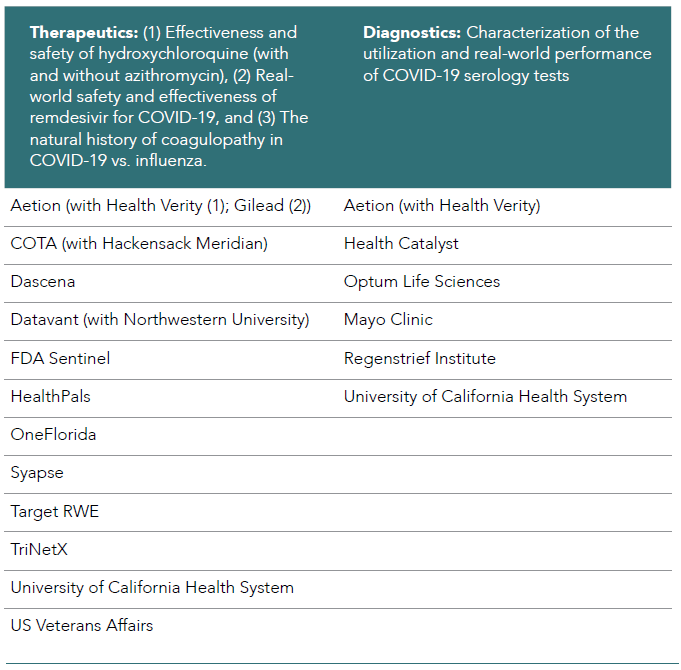
The primary research aims were to identify where test data reside in a data ecosystem, and to connect that data to enable researchers to answer key questions: 1) Among persons with molecularly-confirmed SARS-CoV-2, describe serological testing by demographic, behavioral and environmental characteristics, baseline clinical presentation, key comorbidities, and bacterial/viral co-infections, 2) Characterize the timing of serology testing relative to symptom onset or RNA date by demographic, behavioral and environmental characteristics, baseline clinical presentation, key comorbidities, bacterial/viral co-infections, and test characteristics (e.g., manufacturer), 3) Describe demographic, behavioral and environmental characteristics, baseline clinical presentation, key comorbidities, bacterial/viral co-infections, and test characteristics (e.g., manufacturer) associated with positive serology vs. negative serology, and 4) Describe the real-world positive percent agreement of serology compared to positive molecular test for SARS-COV-2.
The Diagnostics Parallel Analysis workgroup has convened 36 times and presented on 3 occasions to the Diagnostics Lab Meeting to report out results from their analysis to characterize real-world data interoperability, use, and performance of SARS-CoV-2 serology tests. To date, one manuscript is under review and two others are in development.