Author: Li H
Description
Selection bias occurs “when the estimate of occurrence or of etiologic effect obtained from a study population differs systematically from the estimate that would have been obtained had the source population been available.”18 Selection bias can be present when the criteria used to select patients into study cohorts (e.g., exposed and unexposed cohorts) are inherently different. Much of the scientific evidence informing health policy and clinical decision making during the COVID-19 pandemic has been provided from non-interventional, observational studies. Although the evaluation of data obtained from non-interventional studies offers insights into medication effectiveness, caution is required when interpreting results. Selection bias can occur when studies use data sets with selection mechanisms that govern how patients enter the data set or cohort, such as when eligibility is based on patients admitted to the hospital, tested for active infection, or who volunteer to participate in a study, as recently articulated by Griffiths et al.19
Directed acyclic graphs are useful for depicting causal structures and can be used to illustrate common biases, including selection bias. Structurally, selection bias occurs when an investigator conditions on a common effect of 2 or more variables, such as when conditioning on effects of both an exposure (or a descendent of exposure) and an outcome (or a descendent of the outcome). This is sometimes referred to as collider stratification bias, and it induces a statistical relation between the exposure and outcome that is not causal. A structural classification of bias and confounding, illustrated in Figure 3.1, distinguishes between biases resulting from conditioning on common effects (“selection bias”) and those resulting from the existence of common causes of exposure and outcome (“confounding”), which is described above.20 Immediately below are some example studies that have highlighted concerns in selection bias in the setting of COVID-19 real world evidence studies.
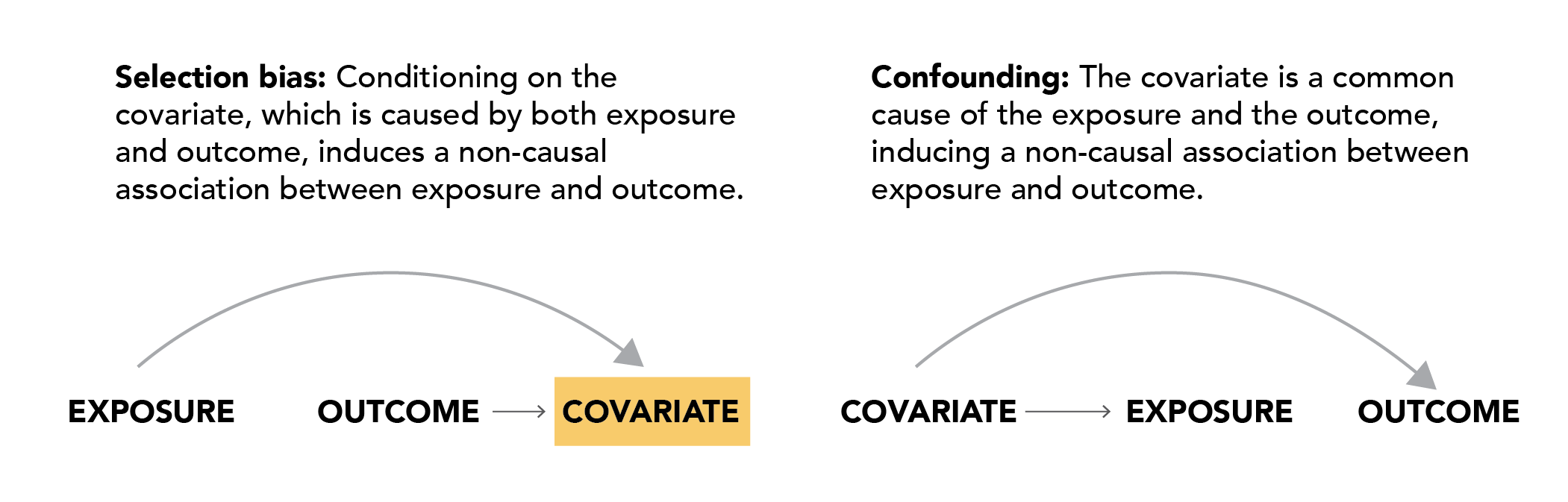
Figure 3.1. Selection bias in the association between outpatient medication collected at hospital admission and outcomes occurring during hospitalization
Several studies have been published to evaluate the association between outpatient medications in relation to SARS-CoV-2 infection, recovery, or mortality using records of outpatient medications collected at hospital admission for COVID-19. These include studies of oral medications including diphenhydramine,21 angiotensin-converting enzyme inhibitors and angiotensin II receptor blockers,22–24 HMG-CoA reductase inhibitors (statins).25 Griffith and colleagues describe several scenarios in which restricting study cohorts to individuals who are hospitalized with COVID-19 or who are tested for SARS-CoV-2 can induce selection bias.19 For example, conditioning on having a SARS-CoV-2 test could induce selection bias in a study that seeks to determine whether cigarette smoking is associated with COVID-19 severity if health care workers are less likely to smoke but more like to be tested for SARS-CoV-2 as compared to non-health care workers. In this situation, SARS-CoV-2 is a potential collider because both being a health care worker and COVID-19 severity are likely to be causes of testing. Therefore, conditioning on SARS-CoV-2 testing would induce a potential statistical, but non-causal, relation between smoking and COVID-19 severity.
Selection bias in the association between inpatient medication use and clinical outcomes during hospitalization
In any observational cohort study aiming to compare 2 or more treatment strategies, selection bias can occur when the criteria for selecting patients into—and particularly excluding patients from— the cohort is different between groups. For example, in one study, exposure was based on receiving hydroxychloroquine26 within 48 hours of hospitalization. Hydroxychloroquine-exposed patients were compared to unexposed patients. Unexposed patients were defined as those who did not initiate hydroxychloroquine at any time during hospitalization; those patients who initiated treatment more than 48 hours after hospital admission were therefore excluded in the primary analysis. Because hydroxychloroquine was as a treatment for severe COVID, excluding from the unexposed group those patients who went on to initiate treatment after the 48-hour exposure assessment window effectively selected out from the unexposed group many patients who deteriorated and went on to have poor outcomes. A similar design was used to compare remdesivir exposure to non-exposure.27 Looking beyond the start of follow-up to exclude patients from the study can introduce selection bias in addition to immortal time bias (see Section 3.3).
Self-selection into studies, missing data, and loss to follow-up are threats to the validity of exposure-outcome association estimates
Prospective studies in which COVID-19 status can be determined through voluntary participation, such as survey data that are linked to administrative records28 or mobile phone-based applications,19 can be subject to selection forces that govern who is included in the study, which can affect the generalizability of study findings. As population testing for COVID-19 is not generally performed in random samples, studies have found that participants who volunteer for scientific studies conditional on having had a test are more likely to be highly educated, health conscious, and non-smokers as compared to the general population.19
Both missing data when selection is based on availability of such data (see Section 3.4) and differential losses to follow-up (i.e., informative censoring) can introduce selection bias. Differential losses to follow-up occur when participants drop out of a study or can no longer be followed for reasons related to the outcome(s) of interest and when this dropout occurs more frequently in more treatment groups.29
Why it is a problem?
Selection bias can compromise the internal validity of a study by distorting the association between exposure and outcomes. Furthermore, collider stratification bias can also be introduced when making statistical adjustments for variables that lie on the causal pathway between an exposure and outcome, when confounders (i.e., common causes) exist between these intermediate variables and the outcome of interest. Independent of this potential form of collider stratification bias, conditioning variables that are intermediates on the causal pathway between exposure and outcome can also obscure the ability to identify a causal effect.
How to handle it
While loss to follow-up, self-selection, and missing data are generally not fully avoidable in real-world data studies, careful study design can reduce these issues and mitigate resulting bias. Researchers can also mitigate selection bias by clearly defining the target population of interest and examining potential impact by implementing sensitivity analyses if they have quantitative knowledge about factors influencing selection in their study.30
In case-control studies, selection bias can be reduced by sampling controls in a manner to ensure that they will represent the exposure distribution in the population that gave rise to the cases. When determinants of selection or censoring are known and measured, inverse probability weighting can be used to address structural selection biases through the creation of sampling weights or censoring weights.31 Approaches to addressing missing data are described below.