Authors: Gatto N, Rassen J
What is it used for?
Between-person observational study designs include cohort studies and case-control studies.36,37
-
Cohort studies (specifically parallel-group cohort studies) compare exposed and unexposed groups of patients over time for the occurrence of the outcome of interest. Cohort study designs have been used to examine the effectiveness and safety of COVID-19 treatments, and can be designed to emulate a hypothetical parallel-group randomized clinical trial.
- Case-control studies differ from cohort studies with respect to patient selection, first identifying patients with the disease or outcome of interest (cases) and then a sample of those without the disease or outcome (controls). Case-control studies compare these cases and controls and examine differences in their preceding exposure status. Case-control studies may be sampled from a corresponding cohort study (i.e., a nested case-control study) or sampled from an underlying source population representing a hypothetical cohort if a full cohort study was performed (i.e., all available controls would have been included, rather than just a sample). Cases and controls are selected that recreate the exposure distribution in the underlying source population.
For the examination of COVID-19 vaccine effectiveness, there is growing interest in using a type of case-control study—the test-negative study design—in which patients with a positive COVID-19 test are considered cases and those with a negative test, controls, and vaccine status is the preceding exposure of interest.38–40 The rationale for this study design is to ensure cases and controls have equal access to health care and health seeking behavior by including only persons with an encounter (here, a COVID-19 test) for surveillance of the outcome. Of note, while this design may help overcome differential outcome misclassification where COVID testing is dependent on certain patient characteristics including vaccination status or symptoms, outcome misclassification may still occur because of low test sensitivity, specificity, and timing of the diagnostic assay.41,42 Thompson et al. conducted a simulation study, reporting that the presence of both exposure and outcome misclassification led to an underestimation of the effectiveness of COVID-19 vaccines.39
Data from health care settings such as primary and specialty care, hospitals, emergency rooms, and urgent care allow access to cases and controls who can then be queried for previous exposures. If longitudinal RWD are available and no primary data ascertainment is required, both nested case-control and cohort studies are feasible. Because nested case-control studies typically provide little advantage or efficiency over conducting cohort studies using existing RWD, and should yield a substantially similar result,43 we focus on study design considerations for cohort studies throughout this section. With that said, case-control designs can be useful if key variables are difficult or expensive to measure, or if nuances of timing between exposure and outcome are of particular interest.
Study Population
For COVID-19, the population of interest often includes patients diagnosed with COVID-19 in the ambulatory outpatient setting (with mild disease) or inpatient setting (with moderate to severe disease). Identifying these analytic cohorts requires careful consideration of several study design parameters (see Table 2.1).
Table 2.1. Key Study Design Parameters
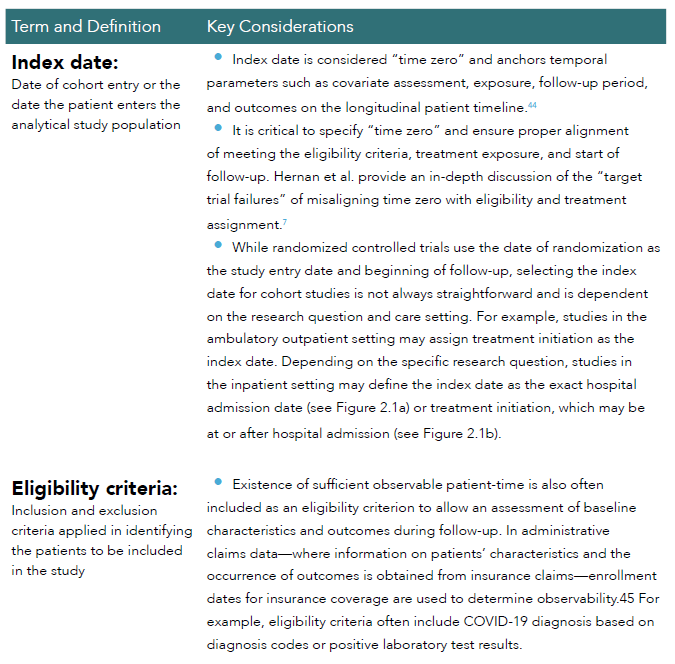
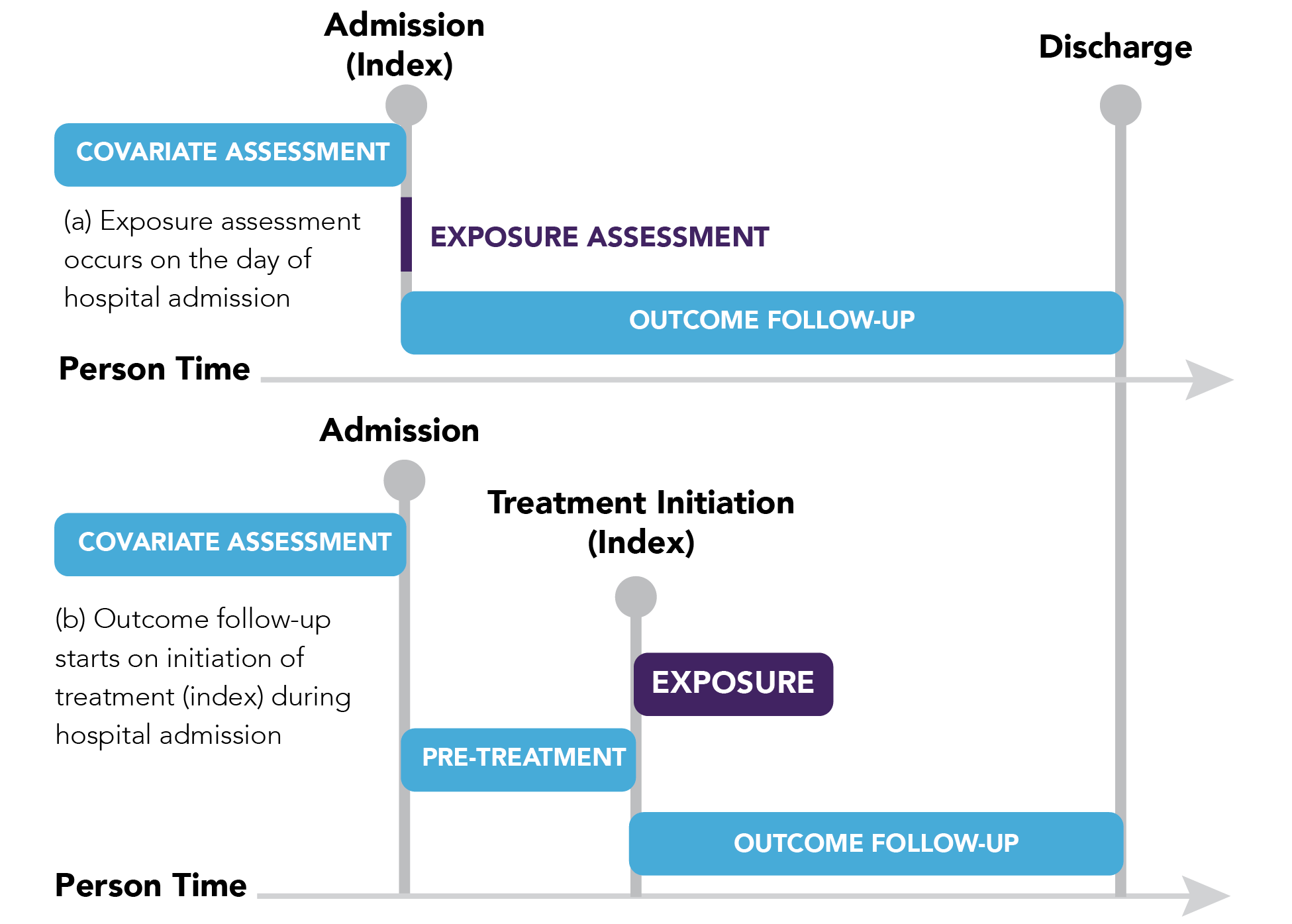
Figure 2.1. Defining the index date for cohort studies in the inpatient setting. Adapted from Franklin et al. Clinical Pharmacology & Therapeutics 2021.48
Treatment Exposure
The new-user design (also known as incident-user design) and prevalent-user design are common approaches for identifying drug exposure (see Figure 2.2). The new-user study design has been previously well-described.34,46,47 Under this design, patients who are new users of a drug of interest—with new use defined as using the drug with no prior use over a prespecified washout period—are compared to patients who are new users of another drug (active comparator) or patients who did not initiate the drug of interest (non-user comparator). The index date for new-user design with an active comparator is typically the day of medication initiation for both groups (see Figure 2.2b). For example, in a study to evaluate whether corticosteroid A reduces the risk of inpatient mortality compared to corticosteroid B, the index date would typically be the date of inpatient treatment initiation of the first corticosteroid.
In contrast, the prevalent-user study design examines both current and new initiators of treatment during the study period.48 The index date for patients in the prevalent-user design would vary. As seen in Figure 2.2c, the index date for patients 4 – 7 only would be treatment initiation, while the index date for patients 1 – 3 would occur at different periods of high-, medium-, and low-risk of the outcome event after treatment initiation depending on the patient. For example, in a study evaluating the effect of medications on COVID-19 severity that are commonly used but not specifically indicated for COVID-19, such as angiotensin converting enzyme (ACE) inhibitors/angiotensin-receptor blocker (ARB), patients may have had prior exposure to the medication for indications other than COVID-19. If so, the new-user design may be deemed inappropriate (or infeasible) in this particular setting and a prevalent-user design may be considered in which all medications used at the time of or shortly following a positive COVID-19 test may be evaluated as the treatment exposure. Patients would then be followed from the time of treatment to ascertain COVID-19-related outcomes.
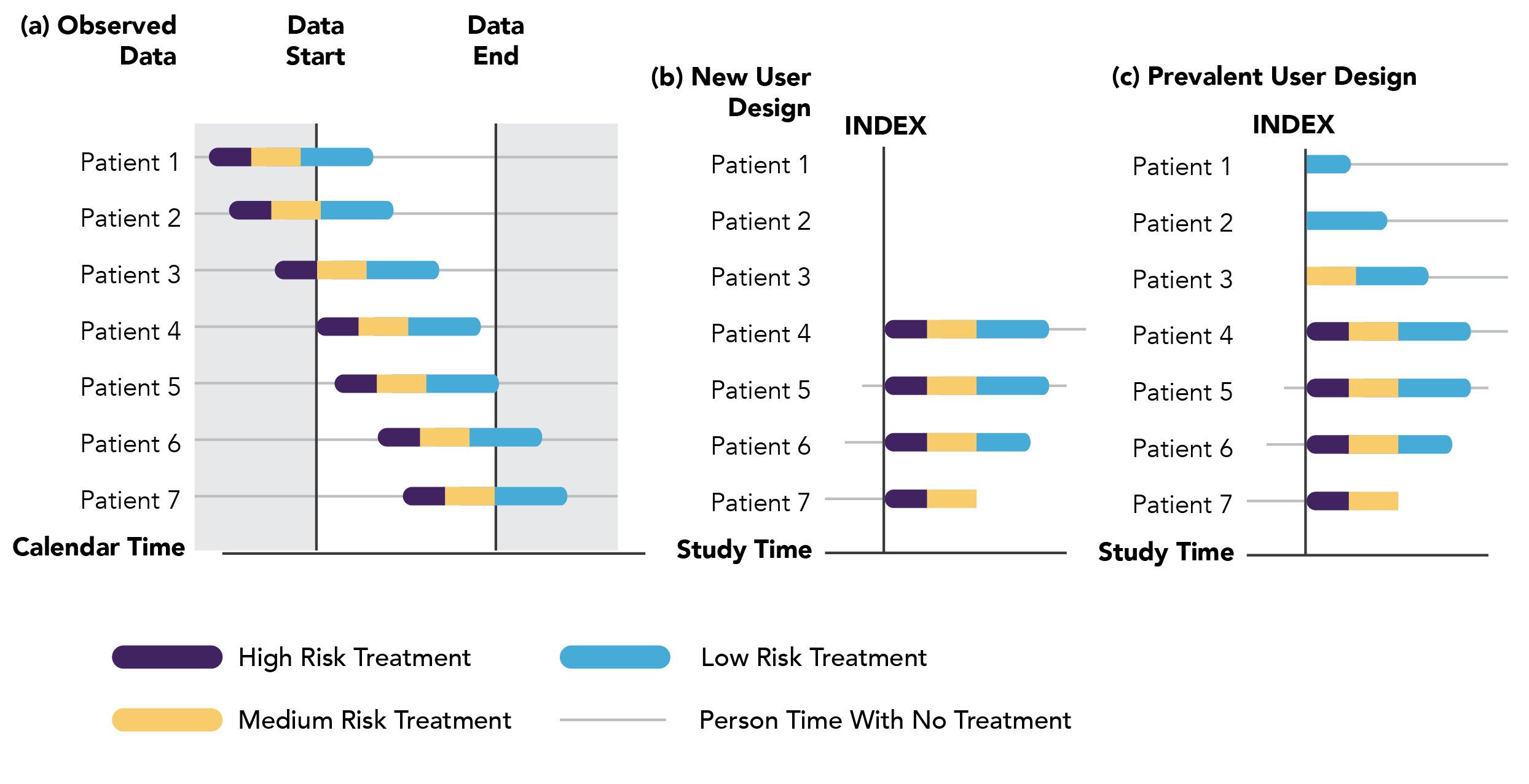
Figure 2.2. New-user and prevalent-user design. Adapted from Yoshida et al. Nat Rev. Rheumatol. 2015.61 (a) Illustrates a hypothetical study cohort during the study period, as represented by the white, unshaded area. The color bars represent treatment and high-, medium-, and low-risk for the outcome event during treatment exposure. The gray line indicates untreated person time. (b) The new user design shows the index date as the date of treatment initiation. The cohort includes only newly treated patients 4–7. (c) The prevalent user design shows a defined index date including all the treated patients 1–7.
More recently, the prevalent new-user design has emerged as another approach to identify exposure to the treatment of interest. A prevalent new-user design creates comparison groups by matching patients on time-based propensity scores, allowing patients who have switched treatments to enter the study.49–51 The prevalent new-user design can result in greater statistical power than conventional new-user designs by allowing all or a majority of exposed patients to enter the study, versus designs that substantially limit the eligible population. Another advantage of this study design is its ability to mimic a randomized controlled trial since both exposed and unexposed patients are matched based on time.52
Choice of Comparator or Control
One of the most critical considerations in designing a cohort study is ensuring balance between the exposure and comparator groups with regard to factors that may influence the outcome, such as calendar time (given the rapidly evolving nature of COVID-19 spread, treatment, and management), disease severity, comorbidities (e.g., diabetes and cardiovascular disease), and race and social determinants of health.48 An active comparator is another medication with a similar indication and is ideal to balance measured characteristics between the two treatment groups and minimize confounding by indication. Paired with the new user design, assuming the two treatment options are clinically interchangeable, an active comparator also minimizes confounding by severity and starts patients at a similar time zero (start of a new treatment), thus allowing emulation of a target trial, with the index date defining the date of treatment initiation for each group.
With rapidly changing treatment regimens for COVID-19, an active comparator may not be possible if there is no standard of care available, or if standard of care is rapidly evolving. Another option is selecting non-users of the treatment of interest, sometimes called “best available care,” i.e., patients with a COVID-19 diagnosis who did not receive the treatment of interest. A major concern about this design is confounding, including confounding by indication if patients receiving and not receiving the treatment are inherently different in unmeasurable ways, as well as prescribing patterns and drug access which may introduce additional unmeasurable bias. Identifying an appropriate index date for the non-user group is also challenging since there is no treatment initiation date to anchor on, and one must avoid using a treatment index date that inadvertently incorporates immortal time into the study design (see Chapter 3 for more on immortal time bias).7,52,53 Employing a risk-set sampling design to identify comparators who have not (yet) initiated the treatment of interest and assigning the same study entry date to that comparator allows exposed patients to be matched with unexposed patients on important factors such as calendar time (e.g., month of hospitalization), disease severity (e.g., need for oxygen support), other relevant medications, etc., at the time each exposed patient initiates their treatment.48,54 For example, Figure 2.3 illustrates a simplified cohort of patients hospitalized for COVID-19 during the study period. Patients 1, 4, and 7 received inpatient treatment for COVID-19 and were risk-set sampled with 1:2 matching to non-users. Non-user patients will be included in the referent comparator group.
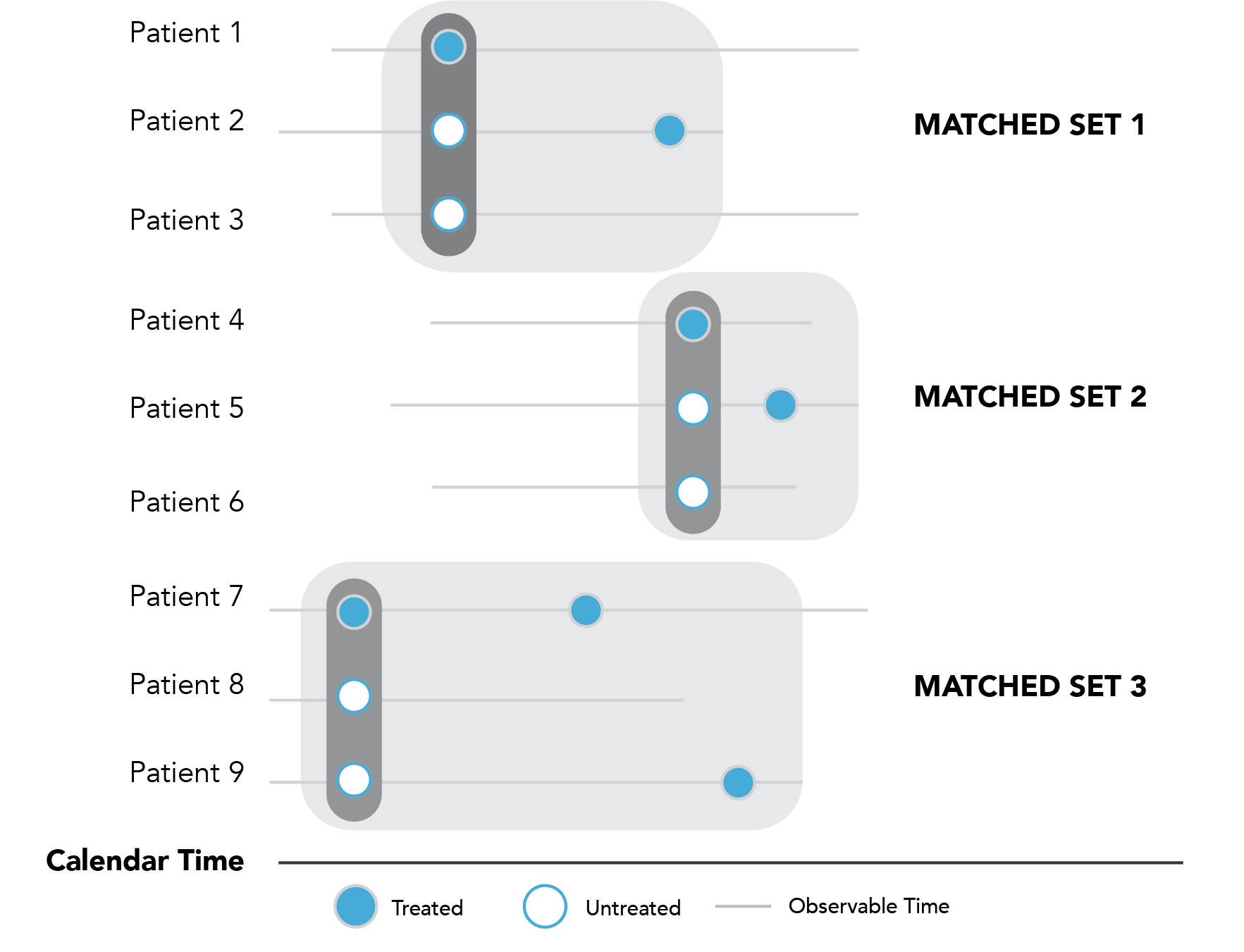
Figure 2.3. Illustrative example of risk-set sampling with users and non-users.
Outcomes and Covariates
For studies using RWD, outcomes are generally defined by applying algorithms to recorded codes, the sensitivity and specificity of which is highly dependent on the type of the data source and data availability. Clinical covariates, such as use of inpatient oxygen support, may also be derived from the data. The importance of clinical expertise to define the case or covariates definitions and develop algorithms cannot be overstated, especially given the rapidly-changing nature of COVID-19 care.
The care setting and point-of-service for COVID-19 is also an important consideration (e.g., outpatient setting or inpatient setting). For example, common inpatient setting-based outcomes when looking at the effectiveness of COVID-19 treatment regimens may include death, use of mechanical ventilation, or hospital discharge; these variables—and in the United States, death in particular—may not always be readily available in commonly-used existing data sources. Best practices also require reporting the performance or validity of case definition algorithms; referencing the positive predictive values, sensitivity, or specificity if available; and providing sensitivity analysis to evaluate the impact of outcome misclassification.55,56 Refer to Section 3.4 for more details of misclassification.
During the outcome assessment (follow-up) period, censoring due to treatment crossover needs to be considered as well.32,57,58 For example, in evaluating the effectiveness of dexamethasone among hospitalized COVID-19 patients for mortality over a 28-day period, follow-up can begin one day after the treatment index and continue until the earliest occurrence of mortality, end of follow-up, or discharge from the hospital. This “initial-treatment” approach approximates the intention-to-treat approach often used in trials and assumes patients continue their initial treatment.25, 26 An “as-treated”-style analysis can also be conducted that censors patients upon treatment changes, and the implications of either approach should be carefully weighted based on the postulated pharmacological pathway (e.g., Should follow-up time without the treatment be counted towards the treatment effect if it is expected that the drug effect ceases shortly after the drug is discontinued?).57
Lastly, the overall study design should be visually communicated to show the temporal relationship between the index date and eligibility criteria, covariate assessments, and follow-up period to assess outcome events.44 Such standardized displays for allowing readers to quickly understand how investigators approached the research problem is particularly important in COVID-19 studies given the volume and variety of research being published.
What kinds of questions can be addressed?
Between-person studies can address effectiveness and safety research questions. For example:
- What is the effectiveness of hydroxychloroquine with or without azithromycin among hospitalized COVID-19 patients on mechanical ventilation, hospital discharge, or in-hospital mortality?59
- Are angiotensin-converting enzyme (ACE) inhibitors and angiotensin-receptor blockers (ARBs) safe among patients diagnosed with COVID-19?60
What are the benefits and limitations of this design?
The advantage of a carefully-controlled cohort study design is the ability to emulate a target trial by specifying time zero to approximate randomization in a trial (i.e., eligibility criteria are met, treatment is assigned, and follow-up period begins) and adjust for potential confounders,3,7 which—in the absence of measurement and selection biases—allows for the determination of causality between the exposure and outcome of interest. As such, the cohort study design is well-suited to examine medication effectiveness and safety in outpatient and inpatient hospitalization settings. Cohort studies also allow multiple outcomes to be examined for a single treatment of interest (for example, primary outcome of mortality and secondary outcomes of hospital discharge).
Cohort study designs may not be well-suited due to insurmountable challenges in identifying appropriate comparison groups or the inability to minimize potential confounding or biases. For example, if the vast majority of the population is vaccinated or potential unmeasured differences between patients choosing and not choosing vaccination exist, a self-controlled study design may be superior.
Key considerations in relation to sources of error related to study designs are covered in Chapter 3.